Want to learn more?
If you or your organization want to learn more about DIA’s Research projects or Think Tanks please contact Science@DIAglobal.org.
Data in Clinical Development
Advancing the uses of AI in biopharmaceutical development
To further understand how Artificial Intelligence impacts therapeutic development, DIA is conducting research to demonstrate its potential.
Phase 1. 2017-2018. PharmaTech
In 2018 DIA embarked on Phase 1 of this project with 8 pharmaceutical partners and Tufts University with a goal to further understanding of current and future uses of AI in biopharmaceutical development. The study demonstrated that AI was being utilized in every major function across healthcare, with the highest use occurring in clinical operations functions, followed by pharmacovigilance, safety, and risk management functions.1 (Figure 4) The study also identified that one major hurdle to AI utilization is the lack of validation, which has led to skepticism of AI and hindered widespread adoption.
Figure 4. Phase 1 highlights: Most common uses of AI across Drug Development functions
Ongoing research! Examining the Current Use and Return Of Investment of Artificial Intelligence Supporting Drug Development
Tufts CSDD, DIA, and a working group study comprised of pharmaceutical and biotechnology companies and contract research organizations have embarked on a study to examine and map the current use of and experience with artificial intelligence (AI) including machine learning and natural language processing (NLP) that support the continuum of drug development. This study updates recent advances and opportunities that are being implemented since a 2019 Tufts CSDD – DIA published study and will help companies prioritize AI investment. The study will identify and benchmark applications of AI to areas within clinical operations and development including study design, site identification and patient recruitment, pharmacovigilance, quality assurance, and clinical monitoring and will explore peer company deployment. Tufts CSDD will also assess the impact of AI-uses on the expected net present value (ENPV) of a typical development program and the return on investment (ROI) of AI/ML use in Phase II and III clinical trials.
Currently Launching! Artificial Intelligence for Adverse Events Reporting and Safety Monitoring: DIA is currently seeking funding partners for this study.
DIA invites your organization to participate in this groundbreaking collaborative study exploring the use of AI and machine learning for adverse event reporting and drug safety monitoring. This research will allow your organization to save time and costs by understanding how an algorithm built on diverse, real-world data was developed; learning successes and limitations in the algorithm development process; gaining insight into responsible AI; and utilizing aspects of the algorithm to reproduce, standardize, and scale AI and ML in signal detection from a myriad of each company’s data sources such as EHRs, RCTs, social media, and claims data by processing and retrieving results in real-time.
- Utilization of Study algorithm/model: Subject to agreement, your organization can utilize the study's algorithm/model for non-commercial purposes.
- Review and comment on manuscripts: You will have the opportunity to review and comment on peer-reviewed manuscripts before submission.
- Acknowledgement as a funding source: Your organization will be acknowledged as a funding source in all resulting publications and presentations.
- Access to methodologies and findings: Gain access to novel methodologies and research findings and collaborate with experts in academia, industry, and regulatory bodies.
The effort will leverage unique EHR data from MedStar Health and Hackensack Meridian Health as the data source for the study. The Georgetown-Lombardi Comprehensive Cancer Center will be participating as the Academic and Clinical partners in the effort.
To learn more about DIA’s Research projects, contact science@diaglobal.org.
Think Tank: In the Shadow of Uncertainty for the Future of Generative AI in Medicines Development: A Collaboration to Illuminate the Way
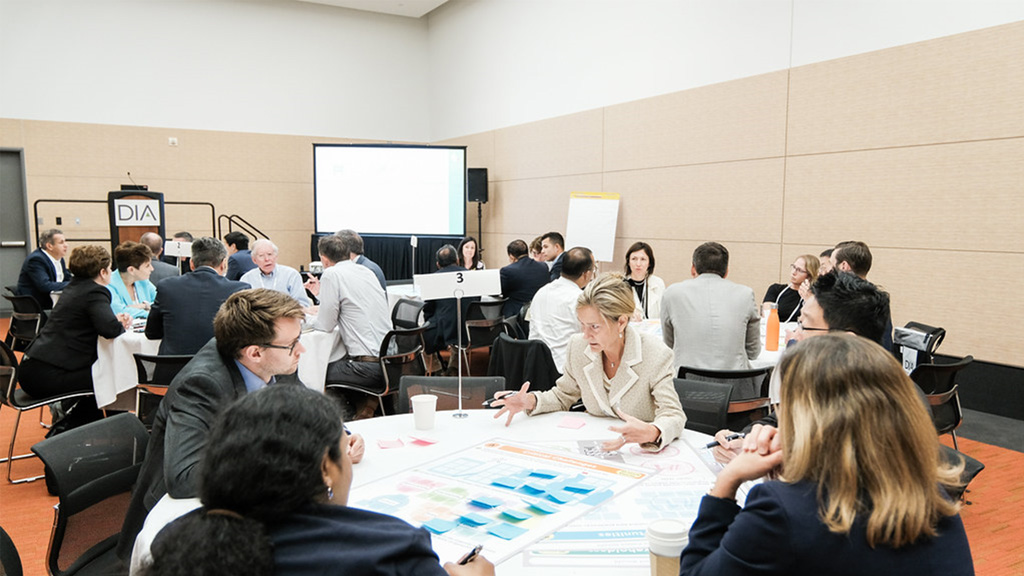
In today's dynamic landscape of medical innovation, convening a diverse array of stakeholders is critical. The transformative potential of generative AI in medicine development is undeniable, but its responsible integration requires a collaborative effort like no other. From researchers and clinicians to regulators, tech innovators, and (ultimately) patients, the convergence of all these voices is the crucible in which we forge a future of healthcare that truly serves everyone. The challenges are myriad. But we can harness the diverse recommendations and best practices of generative AI to revolutionize diagnostics, drug discovery, and patient care through collective dialogue.
At the margins of the DIA Annual Meeting 2023, stakeholders from different regions came together to attend a DIAmond session and participate in a Solution Room focused on Generative AI to discuss and explore the requirements and best practices for implementing generative AI in the pursuit of patient benefit. Through their dynamic exchange of ideas, stakeholders revealed the importance of collaboration as a key to overcoming complex challenges. As we look to the future, one thing becomes abundantly clear: Continued collaborative conversations are not just desirable, they are essential. Through these collaborative forums, transparency is fortified, and partnerships are forged, ultimately laying the groundwork for identifying shared solutions that will shape the future of this groundbreaking field.
For DIAmond Session and Solution Room highlights, please refer to DIA Global Forum
To access the Outcomes report, click here
To learn more about DIA’s Research projects, contact science@diaglobal.org.